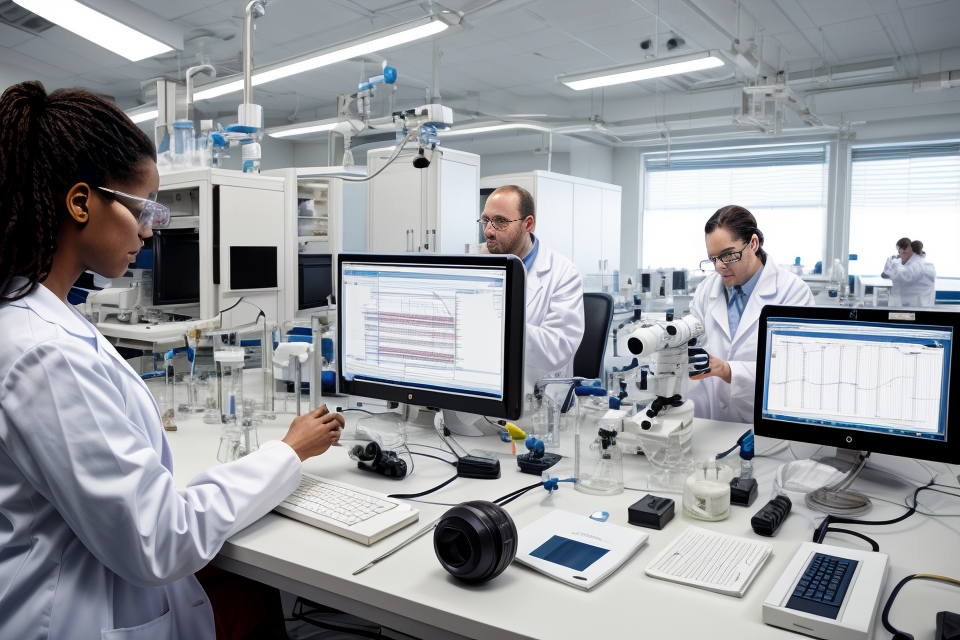
When it comes to data collection, sampling is a widely used technique that involves selecting a subset of individuals or data points from a larger population. But when and where should sampling be applied? In this comprehensive guide, we will explore the different scenarios where sampling can be used effectively to gather meaningful insights. From surveys and experiments to market research and quality control, we will delve into the various applications of sampling techniques and their importance in making informed decisions. Whether you’re a researcher, analyst, or simply curious about data collection, this guide will provide you with a clear understanding of when and where to apply sampling for optimal results. So, let’s dive in and explore the world of effective sampling!
Sampling Techniques: An Overview
Definition of Sampling Techniques
Sampling techniques refer to the methods used to select a subset of individuals or observations from a larger population for the purpose of statistical analysis. These techniques are used to gather data in a more efficient and cost-effective manner, as it is often impractical or impossible to study every member of a population. The results obtained from the sample can then be used to make inferences about the larger population.
Sampling techniques can be broadly classified into two categories: probability sampling and non-probability sampling.
- Probability sampling: In this method, every member of the population has a known, non-zero chance of being selected for the sample. Examples include simple random sampling, stratified random sampling, and cluster sampling.
- Non-probability sampling: In this method, the selection of individuals or observations for the sample is not based on any known probability. Examples include convenience sampling, quota sampling, and snowball sampling.
It is important to choose the appropriate sampling technique based on the research question, the size and characteristics of the population, and the resources available. An inappropriate sampling technique can lead to biased or unreliable results.
Types of Sampling Techniques
When it comes to sampling techniques, there are several methods that can be used depending on the research question, the population, and the sample size. In this section, we will explore the different types of sampling techniques and their applications.
1. Random Sampling
Random sampling is a technique where every member of the population has an equal chance of being selected for the sample. This method is widely used because it is simple, efficient, and provides a representative sample. Random sampling can be done using various methods such as simple random sampling, stratified random sampling, and cluster sampling.
2. Systematic Sampling
Systematic sampling is a method where the researcher selects every nth member of the population. This technique is useful when the population is large and it is not feasible to select every member. Systematic sampling can be done using various methods such as equal-probability sampling and fixed-interval sampling.
3. Snowball Sampling
Snowball sampling is a non-probability sampling technique where the initial sample is chosen based on specific criteria, and then additional samples are recruited based on the responses of the initial sample. This method is useful when the population is hard to identify or when the researcher wants to study a specific subgroup.
4. Volunteer Sampling
Volunteer sampling is a non-probability sampling technique where the sample is composed of individuals who volunteer to participate in the study. This method is useful when the researcher wants to study a specific subgroup or when the population is difficult to access.
5. Convenience Sampling
Convenience sampling is a non-probability sampling technique where the sample is composed of individuals who are easily accessible to the researcher. This method is useful when the researcher has limited time or resources to conduct the study.
6. Purposive Sampling
Purposive sampling is a non-probability sampling technique where the sample is composed of individuals who meet specific criteria. This method is useful when the researcher wants to study a specific subgroup or when the population is difficult to access.
Understanding the different types of sampling techniques is essential for researchers to select the most appropriate method for their study. The choice of sampling technique will depend on the research question, the population, and the sample size.
The Importance of Sampling in Research
Role of Sampling in Data Collection
Sampling plays a critical role in data collection for research purposes. It is a process of selecting a subset of individuals or items from a larger population to represent the entire population. This selection is done based on specific criteria and can be random or stratified.
Sampling is used in research to make the data collection process more efficient and cost-effective. It allows researchers to focus their efforts on a smaller group of individuals or items that are representative of the larger population. This helps to reduce the time and resources required to collect data.
In addition, sampling is used to ensure that the data collected is representative of the population being studied. This is important because if the sample is not representative, the results of the research may not be accurate or generalizable to the larger population.
Moreover, sampling can also help to control for confounding variables that may impact the results of the research. By selecting a specific sample, researchers can ensure that the variables of interest are the focus of the study, and other variables that may influence the results are controlled for.
Overall, sampling is a crucial component of data collection in research. It allows researchers to collect data efficiently and effectively, while ensuring that the data collected is representative of the population being studied.
Factors to Consider When Choosing a Sampling Technique
Choosing the right sampling technique is crucial for obtaining accurate and reliable results in research. Here are some factors to consider when selecting a sampling technique:
- Target Population: The target population is the entire group of individuals or objects that you want to study. For example, if you are conducting a study on the effectiveness of a new medication, the target population would be all patients who are prescribed the medication.
- Sampling Frame: The sampling frame is the complete list of individuals or objects from which you will sample. For example, if you are conducting a study on the eating habits of college students, the sampling frame would be the list of all college students.
- Sample Size: The sample size is the number of individuals or objects that you will select from the sampling frame. The sample size should be large enough to provide accurate results but not so large that it becomes impractical to collect the data.
- Cost: The cost of the sampling technique should be considered, as some techniques may be more expensive than others. For example, random sampling may be more expensive than stratified sampling if the sample size is large.
- Time: The time required to conduct the sampling technique should also be considered. Some techniques may take longer to implement than others, which may impact the overall timeline of the research project.
- Representativeness: The sampling technique should ensure that the sample is representative of the target population. For example, if you are conducting a study on the political views of Americans, you would want to ensure that your sample is representative of the entire population of Americans.
- Validity: The sampling technique should be able to provide valid results. Validity refers to the extent to which the results of the study accurately reflect the true situation.
- Reliability: The sampling technique should be able to provide reliable results. Reliability refers to the extent to which the results of the study are consistent if the study is repeated.
By considering these factors, researchers can select the most appropriate sampling technique for their research project and ensure that they obtain accurate and reliable results.
Choosing the Right Sampling Technique
Determining the Objectives of the Study
Determining the objectives of the study is a crucial step in choosing the right sampling technique. The objectives of the study will determine the type of data that needs to be collected, the population that needs to be studied, and the sampling method that will be most effective.
For example, if the objective of the study is to estimate the mean weight of all adults in a particular city, a simple random sampling technique would be appropriate. However, if the objective of the study is to identify the factors that influence the purchase decision of a particular product, a stratified sampling technique would be more appropriate.
Therefore, it is important to carefully consider the objectives of the study before selecting a sampling technique. This will ensure that the data collected is relevant and useful for achieving the research goals.
Defining the Population and Sample
Defining the population and sample is a crucial step in choosing the right sampling technique. The population refers to the entire group or universe of interest, while the sample is a subset of the population that is selected for study. Defining the population and sample is important because it helps researchers determine the appropriate sampling technique to use, the size of the sample needed, and the characteristics of the sample that is needed.
It is important to consider the characteristics of the population and the research question when defining the population and sample. For example, if the research question is focused on a specific subgroup of the population, then the sample should be selected from that subgroup. Additionally, the characteristics of the population, such as the size and distribution of the population, should be considered when determining the appropriate sampling technique to use.
Another important factor to consider when defining the population and sample is the accessibility of the population. Some populations may be difficult to access or may be spread out over a large geographic area, which can make it difficult to select a representative sample. In these cases, researchers may need to use more sophisticated sampling techniques, such as cluster sampling or multistage sampling, to ensure that the sample is representative of the population.
Overall, defining the population and sample is a critical step in choosing the right sampling technique. It is important to carefully consider the research question, the characteristics of the population, and the accessibility of the population when defining the population and sample.
Considering the Resources Available
When choosing the right sampling technique, it is crucial to consider the resources available. These resources can include time, budget, personnel, and equipment.
Time: The amount of time available for the sampling process can affect the choice of sampling technique. Some techniques, such as random sampling, can be completed quickly, while others, such as stratified sampling, may take more time.
Budget: The budget available for the sampling process can also influence the choice of sampling technique. Some techniques, such as simple random sampling, may be more cost-effective than others, such as cluster sampling, which requires more resources.
Personnel: The availability and expertise of personnel can also impact the choice of sampling technique. For example, if the personnel have advanced statistical knowledge, a more complex sampling technique, such as multistage sampling, may be appropriate.
Equipment: The availability and type of equipment can also play a role in determining the appropriate sampling technique. For instance, if specialized equipment is required, such as in case of environmental sampling, the choice of technique may be limited.
In summary, when choosing the right sampling technique, it is essential to consider the resources available, including time, budget, personnel, and equipment, to ensure that the chosen technique is feasible and effective.
Common Sampling Techniques
Simple Random Sampling
Definition and Explanation
Simple random sampling is a common technique used in research studies to select a representative sample from a larger population. It involves the random selection of individuals or units from a population without any particular bias or discrimination. This technique is considered to be the most basic and unbiased method of sampling.
Procedure
The procedure for simple random sampling involves the following steps:
- Define the population: The first step is to clearly define the population that the study is interested in.
- Determine the sample size: The researcher must determine the size of the sample that will be selected from the population.
- Randomization: The researcher must use a randomization technique to select the sample from the population. This can be done using various methods such as random number generators or tables of random numbers.
- Sample selection: The sample is selected from the population based on the randomization process.
Advantages and Disadvantages
Advantages
- Simple and easy to implement
- Produces a representative sample
- Minimizes bias
- Efficient use of resources
Disadvantages
- Requires a complete list of the population
- Not suitable for small populations
- Cannot be used for studying rare events or rare populations
Conclusion
Simple random sampling is a widely used and reliable technique for selecting a representative sample from a population. It is an unbiased method that produces efficient results with minimal resource usage. However, it has some limitations and may not be suitable for all research studies. Therefore, researchers must carefully consider the advantages and disadvantages of this technique before applying it in their studies.
Stratified Random Sampling
Stratified random sampling is a type of probability sampling technique that involves dividing a population into homogeneous subgroups or strata based on certain characteristics or variables. The goal of stratification is to ensure that each stratum is represented proportionally in the sample, thus ensuring that the sample is representative of the population.
The process of stratified random sampling involves the following steps:
- Identify the population and the variables of interest.
- Divide the population into strata based on the variables of interest.
- Select a sample from each stratum based on simple random sampling.
- Combine the samples from each stratum to form the final sample.
Stratified random sampling is commonly used in social sciences research, such as surveys and political polls, where the population is heterogeneous and complex. It is particularly useful when the researcher wants to ensure that certain subgroups within the population are adequately represented in the sample.
One advantage of stratified random sampling is that it can improve the precision and accuracy of the sample by reducing sampling error. However, it can also be more time-consuming and complex than other sampling techniques.
Overall, stratified random sampling is a useful and effective sampling technique that can help researchers obtain representative and reliable data from complex populations.
Cluster Sampling
Cluster sampling is a technique used in quantitative research to divide a population into smaller groups or clusters and then selecting a sample from these clusters. This method is useful when it is difficult or expensive to collect data from the entire population. In cluster sampling, clusters are selected based on geographical, social, or administrative boundaries.
Cluster sampling can be further divided into two types:
- Stratified Cluster Sampling: In this method, the population is divided into strata or subgroups based on certain characteristics, and then clusters are selected from each stratum. This technique ensures that the sample is representative of the population and helps to reduce sampling error.
- Simple Random Sampling: In this method, clusters are selected randomly from the population. This technique is simple and easy to implement, but it may not be as effective as stratified cluster sampling in terms of representativeness and reducing sampling error.
Cluster sampling has several advantages, including:
- Reduced cost and time required for data collection
- Easier access to hard-to-reach populations
- Increased representativeness of the sample
- Greater control over data collection process
However, cluster sampling also has some disadvantages, including:
- Difficulty in selecting the appropriate number and size of clusters
- Possible selection bias in choosing the clusters
- Limited generalizability of the results to the larger population
In conclusion, cluster sampling is a useful technique in quantitative research when sampling the entire population is difficult or expensive. Researchers should carefully consider the advantages and disadvantages of this method and select the appropriate type of cluster sampling based on their research goals and objectives.
Systematic Sampling
Systematic sampling is a method of selecting a sample from a population by following a specific pattern or sequence. It is a probability-based sampling technique that ensures that each member of the population has an equal chance of being selected for the sample.
In systematic sampling, the researcher selects every nth member of the population, where n is the sampling interval. For example, if the population size is 100 and the sampling interval is 10, then the researcher would select every 10th member of the population.
Systematic sampling is a relatively simple and efficient method of sampling, as it does not require the researcher to have prior knowledge of the population. It is also a cost-effective method, as it allows the researcher to collect data from a large population without having to survey the entire population.
However, systematic sampling may not be appropriate in situations where the population is not well-ordered or where there is no clear pattern or sequence to follow. In such cases, other sampling techniques may be more appropriate.
Overall, systematic sampling is a useful and effective sampling technique that can be applied in a variety of contexts, depending on the research question and the characteristics of the population.
Snowball Sampling
Snowball sampling is a non-probability sampling technique that is commonly used in social and health research. It is particularly useful when studying hard-to-reach populations or those who are difficult to identify.
The technique involves identifying a small number of initial participants (usually called “seeds”) and then recruiting additional participants through referrals from the initial participants. This process continues until the desired sample size is reached.
Advantages of snowball sampling include:
- It can be a cost-effective and time-efficient method of recruiting participants.
- It can be used to study hard-to-reach populations.
- It can provide a diverse sample of participants.
However, there are also some limitations to snowball sampling. One of the main limitations is that it may be difficult to ensure that the sample is representative of the population of interest. Additionally, there may be a bias towards more outgoing or sociable individuals, which could impact the results of the study.
In conclusion, snowball sampling can be a useful technique for recruiting participants in certain situations, but it is important to carefully consider the potential limitations and biases when planning a study.
Advanced Sampling Techniques
Multi-Stage Sampling
Multi-stage sampling is a more complex approach to sampling that involves multiple stages or stages. It is commonly used in large-scale studies, where the population is geographically dispersed or where there are different subgroups within the population. The goal of multi-stage sampling is to increase the precision and representativeness of the sample by selecting a subset of the population that is more likely to be representative of the entire population.
In multi-stage sampling, the sampling process is divided into two or more stages. At each stage, a random sample is drawn from the population. The sampling frame at each stage is different, and the sampling fraction is different. This allows for greater control over the sampling process and helps to ensure that the sample is representative of the population.
There are different types of multi-stage sampling, including:
- Multi-stage cluster sampling: This type of sampling involves dividing the population into clusters or groups, and then selecting a random sample of clusters. Within each cluster, a random sample is drawn. This method is commonly used in studies of health and education, where the population is geographically dispersed.
- Stratified multi-stage sampling: This type of sampling involves dividing the population into strata or subgroups, and then selecting a random sample from each stratum. Within each stratum, a random sample is drawn. This method is commonly used in studies of social and economic issues, where there are different subgroups within the population.
- Probability proportional to size (PPS) multi-stage sampling: This type of sampling involves selecting a random sample of clusters or strata, based on the size of the population within each cluster or stratum. This method is commonly used in studies of business and economics, where the population is large and diverse.
Overall, multi-stage sampling is a powerful tool for increasing the precision and representativeness of the sample. It allows for greater control over the sampling process and helps to ensure that the sample is representative of the population. However, it can also be more complex and time-consuming than other sampling methods. As such, it is important to carefully consider the research question and the characteristics of the population when deciding whether to use multi-stage sampling.
Oversampling and Undersampling
Oversampling and undersampling are two common techniques used in data analysis to address class imbalance issues. These techniques involve manipulating the distribution of data in the dataset to improve the performance of certain machine learning algorithms.
Oversampling
Oversampling is a technique used to balance the class distribution in a dataset by creating additional synthetic samples of the minority class. The idea behind oversampling is to increase the size of the minority class so that it is better represented in the dataset.
There are different approaches to oversampling, including:
- Random oversampling: This involves randomly selecting instances from the minority class and duplicating them multiple times to create new synthetic samples.
- Oversampling with replication: This involves creating new synthetic samples by replicating instances from the minority class.
- Synthetic data generation: This involves generating new synthetic samples using a generator model that mimics the distribution of the minority class.
Undersampling
Undersampling is a technique used to balance the class distribution in a dataset by removing instances from the majority class. The idea behind undersampling is to reduce the size of the majority class so that it is better represented in the dataset.
There are different approaches to undersampling, including:
- Random undersampling: This involves randomly selecting instances from the majority class and removing them from the dataset.
- Undersampling with replication: This involves removing instances from the majority class and replacing them with synthetic samples generated from the minority class.
Choosing between oversampling and undersampling
The choice between oversampling and undersampling depends on the specific problem at hand and the characteristics of the dataset. In general, oversampling is preferred over undersampling because it preserves more information about the minority class and is less likely to discard valuable data. However, oversampling can also lead to overfitting if the synthetic samples are too similar to the original instances.
Undersampling, on the other hand, can lead to loss of information about the majority class and can result in bias towards the minority class. Additionally, undersampling can also lead to overfitting if the synthetic samples are too different from the original instances.
Therefore, it is important to carefully consider the trade-offs between oversampling and undersampling and choose the appropriate technique based on the specific problem and dataset characteristics.
Adaptive Sampling
Adaptive sampling is a technique that allows the sampling rate to change in response to changing conditions. This can be particularly useful in situations where the process being sampled is highly variable or when the goal is to minimize the number of samples required while still obtaining accurate results.
Advantages of Adaptive Sampling
One of the main advantages of adaptive sampling is that it can significantly reduce the number of samples required to obtain accurate results. This can be particularly important in situations where the process being sampled is highly variable or when the cost of sampling is high.
Another advantage of adaptive sampling is that it can help to minimize the effects of measurement noise. By adjusting the sampling rate in response to changing conditions, it is possible to reduce the impact of measurement noise on the final results.
Disadvantages of Adaptive Sampling
One potential disadvantage of adaptive sampling is that it can be more complex to implement than other sampling techniques. This may require additional training or expertise to use effectively.
Another potential disadvantage of adaptive sampling is that it may not be suitable for all types of processes. In some cases, the changing conditions may be too complex or difficult to detect, which could lead to inaccurate results.
Examples of Adaptive Sampling
One example of adaptive sampling is variable-rate sampling, where the sampling rate is adjusted based on the level of variation in the process being sampled. This can be particularly useful in situations where the process is highly variable, such as in process control or quality control.
Another example of adaptive sampling is sequential sampling, where the sampling rate is adjusted based on the results of previous samples. This can be particularly useful in situations where the goal is to detect rare events or to monitor the performance of a process over time.
Overall, adaptive sampling can be a powerful tool for obtaining accurate results while minimizing the number of samples required. However, it may not be suitable for all types of processes, and careful consideration should be given to the specific conditions and goals of the sampling process.
Challenges and Limitations of Sampling
Biases in Sampling
Biases in sampling refer to any systematic error or distortion in the selection of samples that can lead to inaccurate or incomplete representation of the population of interest. There are several types of biases that can occur in sampling, including:
- Sampling bias: This occurs when the sample selected is not representative of the population, due to factors such as non-response, selection bias, or non-coverage. For example, if a survey only reaches out to people who own a certain product, it may not accurately represent the opinions of all consumers.
- Selection bias: This occurs when the process of selecting the sample is itself biased. For example, if a researcher only interviews people who are available during the day, the sample may not accurately represent people who work irregular hours.
- Non-response bias: This occurs when certain groups within the population are less likely to respond to the survey or questionnaire, leading to an underrepresentation of those groups in the sample. For example, if a survey about healthcare only reaches out to people who have access to the internet, it may not accurately represent the opinions of people who do not have access.
- Volunteer bias: This occurs when the people who choose to participate in the study are not representative of the population of interest. For example, if a study about environmental attitudes only attracts people who are already environmentally conscious, it may not accurately represent the opinions of the general population.
It is important to be aware of these biases when designing and conducting a study, as they can significantly impact the validity and reliability of the results. Strategies such as random sampling, stratified sampling, and oversampling can help to reduce biases in sampling.
Sample Size Calculation
Accurate sample size calculation is a crucial aspect of sampling techniques. The sample size determines the representativeness and generalizability of the findings. A small sample size may result in biased or unreliable estimates, while a large sample size may be expensive and time-consuming.
The following are some of the factors that should be considered when calculating the sample size:
- Population size: The larger the population size, the smaller the required sample size.
- Population proportion: The larger the proportion of the population that shares the characteristic of interest, the smaller the required sample size.
- Level of precision: The greater the desired level of precision, the larger the required sample size.
- Margin of error: The smaller the desired margin of error, the larger the required sample size.
There are several statistical methods that can be used to calculate the sample size, including the power analysis and the confidence interval methods. Power analysis is used to determine the sample size required to achieve a certain level of statistical power, while the confidence interval method is used to determine the sample size required to estimate a population parameter within a certain range of values.
It is important to note that the sample size calculation is highly dependent on the research design and the specific research question being asked. Researchers should carefully consider these factors when determining the appropriate sample size for their study.
Non-Response Bias
Non-response bias is a type of bias that occurs when respondents who do not participate in a survey or study differ from those who do participate. This can lead to inaccurate or biased results if the non-responders are systematically different from the responders. Non-response bias can be caused by various factors, such as refusal to participate, failure to follow up, or death.
To minimize non-response bias, it is important to use sampling techniques that maximize response rates and ensure that the sample is representative of the population of interest. For example, stratified sampling, which involves dividing the population into strata or subgroups based on relevant characteristics, can help ensure that the sample is representative of the population. Additionally, follow-up efforts, such as reminders or incentives, can help increase response rates and reduce non-response bias.
It is also important to be aware of potential sources of non-response bias and take steps to address them. For example, if certain groups are more likely to refuse to participate, it may be necessary to oversample those groups to ensure adequate representation. In some cases, it may be necessary to use alternative data sources or methods to supplement the survey data to account for non-response bias.
Best Practices for Effective Sampling
Preparing the Sample Frame
Proper preparation of the sample frame is a critical component of effective sampling techniques. The sample frame is the complete list of all units from which the sample will be drawn. It is essential to ensure that the sample frame is accurate, complete, and up-to-date to avoid any bias or errors in the sampling process. Here are some best practices for preparing the sample frame:
- Define the population: Before preparing the sample frame, it is crucial to define the population that the sample will represent. The population can be defined by geographic location, demographic characteristics, or any other relevant factor.
- Obtain a complete and accurate list: Once the population has been defined, it is necessary to obtain a complete and accurate list of all the units from which the sample will be drawn. This list should include all relevant units, such as individuals, households, or businesses.
- Clean and update the list: The sample frame should be cleaned and updated regularly to ensure that it is accurate and up-to-date. This process involves removing any duplicate entries, correcting any errors, and updating any outdated information.
- Stratify the sample frame: Stratification is the process of dividing the sample frame into smaller groups based on relevant characteristics. This technique helps ensure that the sample is representative of the population and reduces sampling error.
- Randomize the selection process: Randomization is the process of selecting units from the sample frame based on chance. It is essential to randomize the selection process to ensure that the sample is representative of the population and to avoid any bias or errors in the sampling process.
By following these best practices, researchers can ensure that the sample frame is accurate, complete, and up-to-date, which is essential for effective sampling techniques.
Ensuring Data Quality
Effective sampling techniques are essential for obtaining high-quality data that can be used to make informed decisions. To ensure data quality, there are several best practices that researchers should follow:
Proper Training and Education
One of the most critical factors in ensuring data quality is proper training and education. Researchers should have a deep understanding of the sampling techniques they are using and the factors that can affect data quality. They should also be aware of the limitations of their chosen methods and be able to adjust their techniques accordingly.
Standardization of Sampling Procedures
To ensure data quality, it is essential to standardize sampling procedures. This means using consistent methods and protocols across all sampling activities. Standardization helps to minimize human error and ensure that the data collected is accurate and reliable.
Quality Control Measures
Quality control measures are essential for ensuring data quality. Researchers should use a variety of techniques to verify the accuracy of their data, including cross-checking data with multiple sources, conducting statistical analyses, and validating data against known benchmarks.
Proper Data Management
Proper data management is also critical for ensuring data quality. Researchers should store data securely and ensure that it is easily accessible and retrievable. They should also implement strict protocols for data cleaning and transformation to ensure that the data is accurate and complete.
Documentation and Transparency
Finally, researchers should document their sampling procedures and make them transparent to ensure data quality. This includes keeping detailed records of sampling activities, sharing data and methods with other researchers, and being open about any limitations or biases in the data. By following these best practices, researchers can ensure that their data is of high quality and can be used to make informed decisions.
Monitoring Sampling Progress
Effective monitoring of sampling progress is crucial to ensure that the sampling process is on track and producing the desired results. There are several key elements to consider when monitoring sampling progress:
- Sampling rate: It is important to track the sampling rate to ensure that the desired number of samples is being collected within the specified time frame.
- Sample quality: The quality of the samples collected should be regularly assessed to ensure that they meet the requirements for the analysis.
- Sample storage: Proper storage of samples is critical to maintain their integrity and ensure that they can be used for analysis at a later time.
- Sample analysis: Regular analysis of the samples collected can help to identify any issues or potential problems with the sampling process.
By monitoring these key elements, you can ensure that your sampling process is effective and producing accurate results.
Recap of Key Points
- Define the research objectives: Before deciding on a sampling technique, it is crucial to define the research objectives clearly. This will help in selecting the appropriate sampling method that aligns with the research goals.
- Consider the research design: The research design should be taken into account while selecting the sampling technique. The sampling method should be in harmony with the research design to ensure the validity and reliability of the findings.
- Choose the right sample size: The sample size is a critical factor in determining the accuracy of the research findings. It is essential to choose a sample size that is large enough to provide representative results while avoiding unnecessary expenses.
- Diversity is key: A diverse sample is crucial to ensure that the research findings are representative of the population. It is important to ensure that the sample is inclusive of different age groups, genders, ethnicities, and socioeconomic backgrounds.
- Randomization is essential: Randomization is a critical component of sampling as it ensures that the sample is representative of the population. It helps in reducing bias and ensures that the findings are reliable.
- Pilot testing is recommended: Pilot testing is an essential step in the sampling process. It helps in identifying any issues or limitations in the sampling method and provides an opportunity to make necessary adjustments before the actual study.
- Ethical considerations: Ethical considerations should be taken into account while selecting the sampling technique. It is important to ensure that the sampling method does not harm or exploit the participants and that their privacy and confidentiality are maintained.
Future Directions for Sampling Research
Sampling research has come a long way, and there are many exciting developments on the horizon. Here are some future directions for sampling research:
- Advances in technology: The advent of new technologies is revolutionizing the field of sampling. For example, the increasing use of smartphones and other mobile devices has led to the development of new sampling methods that leverage these technologies. Additionally, advances in machine learning and artificial intelligence are allowing researchers to develop more sophisticated sampling strategies that can adapt to changing conditions.
- Increased focus on representativeness: In recent years, there has been a growing recognition of the importance of representativeness in sampling. This means that researchers are increasingly interested in developing sampling methods that produce samples that are representative of the population of interest. This is particularly important in the context of big data, where the sheer volume of data can make it difficult to ensure that the sample is representative.
- Integration of mixed methods: Another area of future research is the integration of mixed methods into sampling. Mixed methods combine qualitative and quantitative methods, and they have the potential to provide a more nuanced understanding of complex phenomena. Researchers are exploring ways to integrate mixed methods into sampling in order to improve the validity and reliability of their findings.
- Ethical considerations: As sampling becomes more widespread, there is a growing need to consider the ethical implications of sampling. This includes issues such as informed consent, privacy, and the potential for harm to participants. Researchers are working to develop ethical guidelines for sampling that take into account these concerns.
- Interdisciplinary approaches: Finally, there is a growing recognition of the importance of interdisciplinary approaches to sampling. This means that researchers from different fields are working together to develop new sampling methods that can be applied across a range of disciplines. This has the potential to lead to more innovative and effective sampling strategies.
FAQs
1. What is sampling and why is it important?
Sampling is the process of selecting a subset of individuals or data points from a larger population in order to make inferences about the population as a whole. It is important because it allows researchers to gather data more efficiently and effectively than if they were to collect data from the entire population. Additionally, sampling can be used to generalize patterns and trends within a population to the population as a whole.
2. What are the different types of sampling methods?
There are several different types of sampling methods, including random sampling, stratified sampling, cluster sampling, and convenience sampling. Random sampling involves selecting individuals or data points from a population at random, while stratified sampling involves dividing a population into subgroups and selecting individuals from each subgroup based on certain criteria. Cluster sampling involves dividing a population into clusters and selecting clusters at random to sample from, while convenience sampling involves selecting individuals or data points that are most easily accessible.
3. When should I use sampling in my research?
Sampling should be used when the population is too large or diverse to study in its entirety, or when collecting data from the entire population would be too costly or time-consuming. Sampling can also be useful when the research question or hypothesis requires a smaller, more focused sample. Additionally, sampling can be used to control for biases or to ensure that the sample is representative of the population.
4. How do I determine the appropriate sample size for my research?
The appropriate sample size for your research will depend on several factors, including the size of the population, the level of precision required, and the margin of error acceptable. In general, a larger sample size will provide more accurate results, but may also be more costly and time-consuming to obtain. It is important to consider these factors, as well as any budget or time constraints, when determining the appropriate sample size for your research.
5. How do I ensure that my sample is representative of the population?
To ensure that your sample is representative of the population, it is important to use a sampling method that is appropriate for the research question and to ensure that the sample is diverse enough to capture the range of experiences and perspectives within the population. Additionally, it may be helpful to use statistical techniques, such as weighting or stratification, to adjust for any biases or imbalances in the sample. Finally, it is important to clearly document the sampling method and any adjustments made to the sample in order to ensure transparency and replicability.